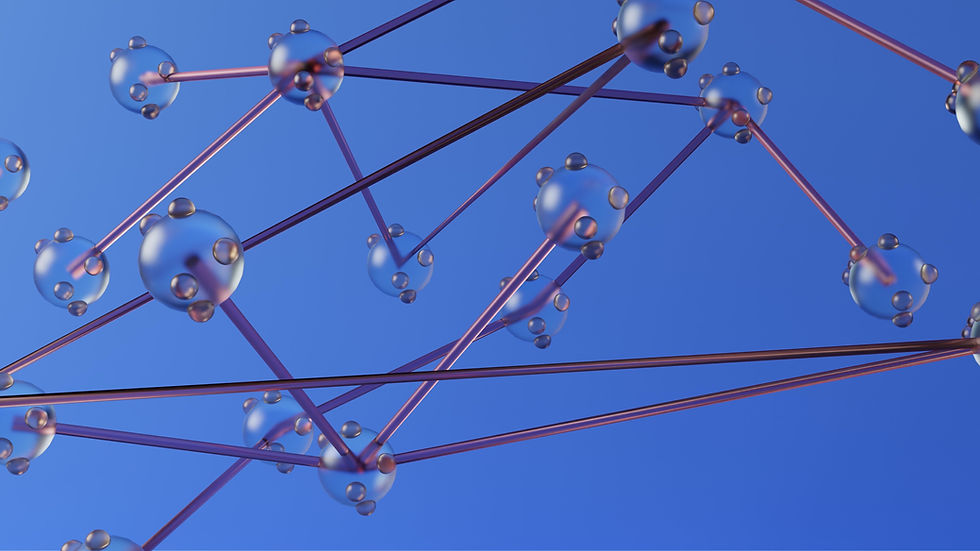
The age-old adage, "Two heads are better than one" best describes this latest development in generative AI technology and here are they key takeaways:
MIT's Computer Science and Artificial Intelligence Laboratory (CSAIL) has introduced a new method that utilizes multiple language models to discuss and debate among themselves to find the best possible answer to a question.
This approach aims to improve the factual accuracy and decision-making of large language models (LLMs) by reducing inconsistencies and flaws in their responses.
The technique involves multiple rounds of response generation and critique. Each model generates an answer and then refines it based on feedback from other agents.
A majority vote across the models' solutions determines the final output, mimicking the dynamics of a group discussion among humans.
The approach can be applied to existing "black-box" models without needing access to their internal workings, making it easy to implement across various LLMs.
In tests, the multi-agent method significantly improved performance in mathematical problem-solving tasks for grade-school and middle/high school math problems.
The approach also reduced the issue of "hallucinations," where language models generate incorrect or random information, by creating an environment that prioritizes factual accuracy.
Beyond language models, the method can be used for integrating different AI models with specialized capabilities in areas like speech, video, or text.
Challenges remain, such as processing very long contexts and refining the models' critique abilities. Further research will explore more complex forms of discussion and collective decision-making.
Yilun Du, an MIT PhD student and the lead author of the research, suggests that this method provides a scalable approach for language models to autonomously improve their factuality and reasoning, reducing reliance on human feedback.
コメント